Ai How To Use
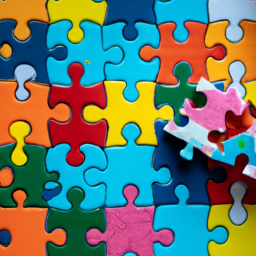
Alright, so you’ve got your hands on a brand new Ai and you’re wondering how to make the most out of it. Well, you’re in luck! In this article, we’ll be sharing some essential tips and tricks on Ai usage. Whether you’re a beginner or just looking to enhance your skills, we’ve got you covered. So sit back, relax, and let’s unlock the full potential of your Ai together!
Understanding the Basics of AI
Artificial Intelligence (AI) is a branch of computer science that focuses on creating intelligent machines capable of mimicking human behavior and performing tasks that typically require human intelligence. It involves the development of algorithms and models that allow computers to learn from and make decisions based on data. AI systems can analyze vast amounts of information, recognize patterns, and make predictions or decisions without explicit human intervention.
Defining AI
AI can be defined as the ability of machines to exhibit human-like intelligence, including the capacity to reason, plan, learn, understand natural language, and perceive and interact with the environment. It encompasses various subfields such as machine learning, natural language processing, computer vision, and robotics.
Key Components of AI
The key components of AI systems include:
- Data: AI heavily relies on extensive and high-quality data to train models and make accurate predictions or decisions.
- Algorithms: AI algorithms serve as the brain behind intelligent systems. They process data, identify patterns, and generate insights or actions.
- Models: AI models are created using algorithms and trained on data to make predictions, solve problems, or perform specific tasks.
- Hardware: The hardware infrastructure, such as powerful processors and storage devices, enables AI systems to process data and execute computations efficiently.
- Software: AI software frameworks provide the tools and libraries necessary for building and deploying AI models and applications.
Different Types of AI
There are mainly two types of AI:
- Narrow AI: Narrow AI, also known as weak AI, focuses on performing specific tasks with high accuracy. Examples include voice assistants like Siri, chatbots, or recommendation systems used by online platforms.
- General AI: General AI, also known as strong AI or artificial general intelligence (AGI), refers to AI systems with human-level intelligence across various domains. Although general AI is still a theoretical concept, it aims to replicate human cognitive abilities and exhibit flexibility similar to humans.
How AI Works
AI systems typically follow a four-step process:
- Data Acquisition: AI systems gather and collect data from various sources, such as databases, sensors, or the internet.
- Data Preparation: The collected data is preprocessed and cleaned to remove any irrelevant or noisy information. This step ensures the data is in a format suitable for training the AI models.
- Model Development: AI models are developed using algorithms and trained on the preprocessed data. The model learns patterns and relationships within the data to make predictions or decisions.
- Deployment: Once the AI model is trained and tested, it is deployed in a real-world environment. The model can then process new data and provide insights, recommendations, or automate tasks.
Applications of AI
AI has found numerous applications across various industries. Let’s explore some of the most significant areas where AI is making a difference.
AI in Healthcare
AI is revolutionizing healthcare by enhancing diagnosis accuracy, predicting patient outcomes, and improving personalized treatment plans. Machine learning algorithms can analyze medical imaging data to detect diseases like cancer at an early stage. AI-powered chatbots and virtual assistants can also offer preliminary medical advice and support patient engagement.
AI in Business
AI provides valuable insights for businesses, enabling them to make data-driven decisions. It helps automate repetitive tasks, optimize processes, and improve operational efficiency. AI-powered chatbots and virtual assistants can handle customer inquiries and provide personalized recommendations, enhancing the customer experience. Additionally, AI algorithms can analyze customer data to identify patterns and preferences, enabling targeted marketing campaigns.
AI in Entertainment
AI is transforming the entertainment industry by revolutionizing content creation, recommendation systems, and personalized experiences. AI algorithms can generate realistic virtual characters for movies and video games, reducing production costs and enhancing visual effects. Recommendation systems powered by AI can suggest movies, music, or books based on users’ preferences, enhancing user engagement and satisfaction.
AI in Transportation
AI plays a vital role in the transportation industry, particularly in the development of autonomous vehicles. Self-driving cars rely on AI algorithms to perceive the environment, make real-time decisions, and navigate safely. AI also helps optimize traffic management, reduce congestion, and improve transportation logistics, leading to more efficient and sustainable transportation systems.
AI in Education
AI is transforming education by creating personalized learning experiences, automating administrative tasks, and facilitating adaptive assessments. AI-powered tutoring systems can adapt to the individual needs and learning pace of students, providing targeted feedback and support. Intelligent chatbots can assist students with their queries, offer learning recommendations, and provide 24/7 access to educational resources.
Benefits of Integrating AI
Integrating AI into various domains offers several benefits that can significantly impact businesses and society as a whole.
Improving Efficiency
AI automation of tasks can significantly improve efficiency and productivity. Machines equipped with AI can analyze vast amounts of data much faster than humans, reducing the time required for decision-making. AI systems can also automate repetitive or mundane tasks, freeing up human resources to focus on more critical and complex activities.
Enhancing Customer Experience
AI can enhance the customer experience by providing personalized recommendations, real-time support, and faster response times. AI-powered chatbots can understand customer inquiries, provide relevant information, and resolve issues without the need for human intervention. This improves customer satisfaction, increases engagement, and fosters loyalty.
Predicting Outcomes
AI algorithms can analyze historical data, identify patterns, and make predictions about future outcomes. This is particularly valuable in industries such as finance, where AI can help predict market trends, stock prices, or risk assessments. Predictive analytics enabled by AI can assist businesses in making informed decisions and strategic planning.
Reducing Human Error
Human error can have severe consequences in many fields, such as healthcare or finance. AI systems can minimize the risk of errors by accurately analyzing data, identifying anomalies, and providing precise insights. This can lead to improved reliability, safety, and decision-making across various domains.
Potential Risks and Downsides of AI
While AI brings numerous benefits, it also poses certain risks and downsides that need to be addressed.
Job Displacement
AI automation and the rise of intelligent machines may lead to job displacement, particularly in industries with repetitive or manual tasks. However, it is essential to recognize that AI also creates new job opportunities by requiring personnel for developing, managing, and deploying AI systems. Upskilling and reskilling the workforce can help mitigate the negative impact of job displacement.
Privacy Concerns
AI relies on vast amounts of data, which raises concerns about privacy and data security. As AI systems generate insights and predictions based on personal data, protecting individuals’ privacy becomes crucial. Strict regulations and guidelines should be in place to ensure the responsible and ethical use of data in AI applications.
AI Bias
AI algorithms learn from data, and if the data used for training is biased, it can lead to biased outcomes or decisions. Bias can arise from historical data that reflects societal prejudices or systemic biases. Efforts should be made to address and mitigate bias through careful data selection, preprocessing, and ongoing monitoring of AI systems.
Misuse of AI Technology
AI technology, if misused or in the wrong hands, can pose significant risks. Cybersecurity threats, misuse of personal data, or AI-powered autonomous weapons are some examples of potential misuse. Regulations, ethical frameworks, and monitoring mechanisms should be in place to ensure responsible and accountable use of AI technology.
Ethical Considerations in AI Implementation
Implementing AI comes with ethical considerations that need to be addressed to ensure responsible and fair use of AI technology.
Ensuring Transparency
AI systems should be transparent and explainable to build trust and accountability. Users and stakeholders should understand how decisions are made and the factors influencing AI predictions or actions. This transparency allows for auditing, addressing biases, and mitigating potential risks.
Bias and Discrimination
AI algorithms can inadvertently perpetuate bias or discrimination present in historical data. Efforts should be made to identify and correct biases, ensuring that AI systems treat individuals fairly and do not discriminate based on characteristics such as race, gender, or ethnicity. Regular audits and ongoing monitoring are crucial for detecting and addressing biases.
Maintaining Privacy and Security
As AI systems heavily rely on personal data, privacy and security become paramount. Organizations must ensure that data collection, storage, and processing comply with relevant data protection regulations. Robust security measures should be implemented to protect data from unauthorized access or breaches.
Regulatory Compliance
AI implementation should adhere to legal and regulatory requirements. Governments and regulatory bodies play a crucial role in setting standards, guidelines, and frameworks to govern the ethical use of AI technology. Organizations must comply with these regulations to ensure responsible use and mitigate potential risks.
AI Usage: Step-by-Step Guide
Implementing AI in an organization or any other setting involves several fundamental steps. Let’s explore an overview of the typical process.
Identifying a Problem to Solve With AI
The first step in using AI is to identify a specific problem or challenge that AI can help solve. This could be automating a repetitive task, improving decision-making, or enhancing customer experiences. Clearly defining the problem allows for understanding the specific requirements and desired outcomes from an AI system.
Data Collection and Cleaning
AI heavily relies on data, so collecting relevant and high-quality data is crucial. Data can be sourced from various internal and external sources, such as databases, sensors, or online platforms. Once data is collected, it needs to be cleaned and preprocessed to remove any inconsistencies, duplicates, or irrelevant information that might affect the performance of AI models.
Developing and Training AI Models
AI models are developed using algorithms that suit the problem at hand. Depending on the problem type, machine learning algorithms such as supervised learning, unsupervised learning, or reinforcement learning can be employed. The AI models are then trained on the preprocessed data by exposing them to a large quantity of labeled or unlabeled data, depending on the algorithm type.
Deploying and Monitoring AI Systems
Once the AI models are trained and tested, they are ready to be deployed in a real-world environment. This involves integrating the AI system into existing systems or developing new systems and infrastructure to leverage AI capabilities. It is crucial to monitor the performance and behavior of AI systems continuously, ensuring that they operate as intended and make accurate predictions or decisions.
Deciding on AI Tools
When implementing AI, choosing the right AI tools is essential to meet the specific requirements of the problem at hand.
Types of AI Tools
AI tools encompass various frameworks, libraries, and software platforms that support the development, deployment, and management of AI models. Machine learning frameworks like TensorFlow, PyTorch, or scikit-learn provide the necessary tools for building AI models. Natural language processing libraries like NLTK or spaCy enable the processing and understanding of human language. Additionally, cloud-based AI platforms like Google Cloud AI or Azure Machine Learning provide infrastructure and services for scalable AI applications.
Choosing the Right AI Tool
Choosing the right AI tool depends on factors such as the problem type, available resources, and expertise in AI technologies. It is essential to assess the features, capabilities, and compatibility of the AI tools to ensure they align with the requirements and objectives of the AI project.
Understanding AI Tool Capabilities
Different AI tools have varying capabilities and strengths. Some tools might excel in image recognition tasks, while others might be better suited for natural language processing or time-series analysis. Understanding the capabilities and limitations of AI tools helps in selecting the most suitable tool for a specific use case.
Incorporating AI Tools into Existing Systems
Integrating AI tools into existing systems requires careful planning and development. It involves connecting AI models with data sources, establishing data pipelines, and developing APIs or interfaces for seamless interaction between AI systems and other components of the organization’s infrastructure.
Training and Testing AI Models
The process of training and testing AI models is crucial to ensure they perform accurately and reliably.
Understanding AI Training
Training AI models involves exposing them to a large quantity of labeled or unlabeled data. The models learn patterns and relationships within the data through iterative processes, adjusting their internal parameters to improve performance. The accuracy and quality of the training data significantly impact the model’s ability to make accurate predictions or decisions.
Methods of AI Testing
Testing AI models involves evaluating their performance on unseen data. Various testing methodologies, such as cross-validation or holdout validation, can be used to assess model performance. Testing should cover different scenarios and edge cases to understand the model’s behavior in real-world situations.
Refining and Retraining Models
AI models may require refinement and retraining to improve their accuracy or address issues such as biases or errors. Analyzing the model’s performance, receiving feedback from users, and monitoring its behavior in the deployed environment can help identify areas for improvement. Models can be retrained using updated or additional data to enhance their performance.
Interpreting Test Results
Interpreting test results involves analyzing metrics such as accuracy, precision, recall, or F1 score to assess the model’s performance. Understanding these metrics and their implications helps in identifying areas for improvement and optimizing the model’s performance.
AI Adoption Challenges
While AI offers significant potential, its adoption comes with challenges and hurdles that organizations need to overcome.
Data Accessibility and Quality Issues
Accessing relevant and high-quality data can be a significant challenge. Inadequate or incomplete data can lead to biased or inaccurate AI models. Organizations must invest in data collection, management, and quality assurance processes to ensure the availability of reliable data for training AI models.
Lack of Skilled Personnel
The shortage of skilled AI professionals and experts can hinder AI adoption. Building and deploying AI systems require expertise in data science, machine learning, and software engineering. Organizations need to invest in training their existing workforce or hiring specialized talent to navigate and harness the power of AI effectively.
Cost of Implementation
Implementing AI systems can involve significant upfront costs, including infrastructure, tools, and personnel. Organizations need to evaluate the return on investment and carefully plan the implementation to ensure cost-effectiveness. Collaboration with AI vendors or leveraging cloud-based AI platforms can help reduce costs and minimize the initial investment.
Change Management Challenges
Implementing AI often requires organizational and cultural changes. Employees may face resistance or fear due to job displacement concerns or changes in job roles. Change management strategies, effective communication, and training programs are necessary to mitigate resistance and foster a smooth transition to AI-driven processes.
Future Trends in AI
AI continues to evolve rapidly, and several trends are shaping its future.
AI and Big Data
The increasing volumes of data generated by individuals, organizations, and connected devices provide vast opportunities for AI. AI systems can leverage big data to gain deeper insights, improve predictions, and enhance decision-making. The convergence of AI and big data enables organizations to unlock new possibilities, innovate, and create value.
AI in Cybersecurity
As cyber threats become more sophisticated, AI plays a crucial role in enhancing cybersecurity capabilities. AI-powered systems can detect anomalies, analyze network traffic, and identify potential security breaches in real-time. AI-based intrusion detection and prevention systems can significantly strengthen cybersecurity defenses, protecting organizations from evolving threats.
AI in Quantum Computing
Quantum computing has the potential to revolutionize AI by exponentially enhancing computational power. Quantum computers can solve complex optimization problems and process massive amounts of data at a speed that surpasses classical computers. This convergence of AI and quantum computing holds great promise for breakthroughs in various fields, including drug discovery, financial modeling, and climate research.
The Role of AI in Sustainable Development
AI can contribute significantly to sustainable development by optimizing resource allocation, improving energy efficiency, and facilitating green initiatives. AI systems can analyze data to identify opportunities for energy savings, optimize transportation logistics to reduce emissions, or predict environmental risks. Integrating AI into sustainable development strategies enables data-driven decision-making and promotes a more sustainable and resilient future.
In conclusion, understanding the basics of AI is crucial for individuals and organizations looking to leverage the power of AI. From its definition and key components to different types and applications, AI offers tremendous potential to transform various industries. The benefits of integrating AI range from improving efficiency and enhancing customer experience to predicting outcomes and reducing human error. However, it is important to consider the potential risks and downsides of AI, such as job displacement, privacy concerns, AI bias, and misuse of technology. Implementing AI requires careful planning, addressing ethical considerations, selecting the right AI tools, training and testing AI models, overcoming adoption challenges, and preparing for future trends. By embracing AI responsibly, organizations can unlock its full potential and drive innovation and productivity in an increasingly AI-driven world.